As we stand in the present, we often look back to understand the ingenious mechanics behind baseball’s statistical framework. The Earned Run Average (ERA), a pivotal stat in the game, quantifies a pitcher’s excellence with deceptive simplicity. The methodology for calculating ERA has evolved significantly, marked by various pivotal points in baseball’s cherished history. For decades, statisticians and baseball aficionados have refined the ERA, adding depth and nuance to this critical measurement.
“7 Key Historical ERA Calculation Methods Explored” offers an insightful expedition through the chronology of ERA’s calculation. Here, we reveal how initial methods rooted in mere innings and runs have morphed into sophisticated systems that consider nuanced game situations and pitcher-specific contexts. By spotlighting these methodologies, we underscore not just their mathematical progression, but also the evolving intelligence of a game deeply intertwined with America’s cultural fabric.
As we delve into the sophisticated journey from basic innings and runs to intricate statistical models, the Evolution of ERA Calculation Techniques article stands out as a pivotal resource. It meticulously outlines the gradual sophistication of ERA metrics, emphasizing the technological and analytical advancements that have shaped modern baseball analytics.
7 Key Historical ERA Calculation Methods Explored
The Inception of ERA: Simple Innings and Runs
The concept of ERA was initially straightforward. Pitchers were judged by how many runs they allowed per game, a method that reflected the era’s simplicity in data gathering. However, the definition of a ‘run’ was less nuanced than today, and the recording of innings pitched varied considerably. Henry Chadwick, a pioneer in baseball statistics, is credited with the creation of ERA in the 19th century. By accounting for innings pitched over a standard game length of nine innings, Chadwick’s method sought to provide a clearer picture of a pitcher’s effectiveness. This framework, emphasizing earned runs—those for which the pitcher is statistically responsible— set the stage for all future calculations.

For those interested in how the calculation of pitchers’ effectiveness began, Early Methods for Calculating ERA in Baseball reveals the rudimentary approaches that set the stage for all future enhancements. This article sheds light on the initial formulas that relied heavily on innings pitched and earned runs.
However, the rudimentary nature of Chadwick’s design meant it could not account for the complexities of modern baseballs, such as relief pitching and unearned runs caused by fielding errors. As baseball progressed into the 20th century, the need for more sophisticated analytics became apparent. 1920 marks a pivotal year in ERA’s history as it was then, during the pre-Lively Ball Era, that baseball officials decided to officially adopt ERA as an official statistic. The game had begun to expand rapidly, and the strategic intricacies evolved, demanding a more detailed metric to capture a pitcher’s performance nuances. Relief pitchers began to emerge as a tactical element in later innings, further complicating ERA’s computation.
In the early 20th century, baseball statistics began to evolve with pioneers like John Heydler, who introduced Heydler’s statistics, which eventually contributed to the sophisticated means of evaluating pitchers. Reflecting on origins, the American League (AL) and the National League (NL), including the Negro League, each developed unique statistical hierarchies. Players like Lefty Grove in the AL and Dazzy Vance in the NL showcased striking disparities revealing that RA (Runs Allowed) apportionment was necessary to fairly compare the performances across different ballparks. Statistical aficionados would turn to baseball encyclopedias to delve into the careers of legendary names like Charley Hall and James Otis Crandall, while batters faced a relentless barrage of pitches that would soon be quantified into comprehensive datasets. These metrics have paved the way for sabermetrics to offer a profound narrative on baseball statistics, enhancing our appreciation for the game’s most skilled players.
By the mid-20th century, ERA had been refined to more accurately reflect a pitcher’s skill by considering the fielder’s influence. Advances in recording technology and statistical methods led to a more sophisticated way of distinguishing between earned and unearned runs. This was an era when pitchers often pitched complete games, which meant the calculation of ERA did not need to consider the frequent switches seen in today’s game. As such, while the initial method laid the groundwork for ERA, its evolution was inevitable. The game’s strategists and statisticians recognized that for ERA to remain relevant, it had to adapt to the ever-growing strategies and player roles, highlighting the dynamic adaptation of baseball’s analytical tools to its changing landscape.
The foundational aspects of ERA and its significance in the realm of baseball are thoroughly examined in the Origins of ERA Calculation in Baseball. This piece offers a historical perspective on how ERA emerged as a critical measure of pitching performance, highlighting the contributions of pioneering figures like Henry Chadwick.
The Pre-Modern Era: Accounting for Defensive Errors
Transitioning from the era of complete games and the dominance of starting pitchers, baseball needed to address the gray area presented by defensive errors. Defensive errors, which were previously overlooked, now play a crucial role in shaping a pitcher’s ERA. The distinction between earned and unearned runs became a focal point, as statisticians sought to isolate a pitcher’s accountability from the team’s defensive mishaps. The formula began to incorporate defensive error data, a change supported by improvements in game tracking and record-keeping. This period marked the recognition of the team dynamic in individual statistics, sharpening the accuracy of ERA calculations.
The Historical Development of ERA Calculation provides an insightful overview of the milestones in ERA’s evolution. It charts the course from the simple methods of the early days to the complex formulas used today, illustrating how changes in the game and its strategies necessitated these advancements.
By mid-century, this enhanced formula for ERA calculation took into account the number of outs recorded while a pitcher was in the game, alongside the total number of defensive errors. This was pivotal in creating a fairer assessment of pitchers, contributing to the metric’s integrity. Interesting to note is that during the 1945 MLB season, the league average ERA was 2.98, reflective of the pitcher-dominant era just before the significant offensive surge known as the live-ball era. A pitcher’s ability to maintain a low ERA was highly regarded, as the sharp delineation between earned and unearned runs helped to filter the impact of defensive performances on a pitcher’s statistical record.
Exploring the transitions in ERA calculation, Historic Approaches to Determine ERA in Baseball navigates through the various methodologies that have been employed over the years. It emphasizes how the definition and computation of earned runs have evolved in response to the game’s changing dynamics.
In the later part of the century, as the role of relief pitchers became more prominent, ERA calculations had to again adapt. The onset of specialized statistics for relievers, such as the save, introduced in 1969, and hold, quantified in various ways before its standardization, bespoke the continuing refinement of pitching analytics. Rule changes about the scoring of earned runs when a relief pitcher enters the game also had a significant impact, leading to era-specific calculation adjustments. By the end of the 20th century, the average ERA had risen, with the 1999 season reaching an average of 4.71, showcasing a shift towards stronger offensive play and multiple pitcher utilization within games. The ERA calculation had to be dynamic enough to reflect the strategies encompassing the use of both starters and relievers, illustrating the fluid nature of baseball’s analytical evolution.
The Influence of Technology: ERA in the Statistical Boom
As the Digital Age dawned, baseball analytics underwent an extraordinary transformation, deeply influencing the ERA and its relevancy. The introduction of sabermetrics—the empirical analysis of baseball through statistics—ushered in a new era. This approach, popularized by Bill James in the 1970s and 1980s, leveraged increasingly available computational power to dissect and understand the game in ways never before possible. The advent of PitchFx in 2006 and its successor Statcast, introduced in 2015, have revolutionized the granularity of pitching data available, analyzing aspects like pitch velocity, spin rate, and release point—factors that significantly influence a pitcher’s ERA but had been previously unquantifiable.

The incorporation of technology in baseball has not only enhanced the way performance is measured but also how strategies are developed. Teams began employing data-driven decisions to maximize player and game strategy efficiency, directly impacting how pitchers are utilized and, consequently, how their ERA is interpreted. For instance, the increasing reliance on bullpen specialization—where pitchers are chosen for specific game situations—has altered the traditional calculation of ERA. Teams armed with statistical insights could deploy a left-handed specialist from the bullpen to face a tough left-handed hitter in a high-leverage situation, which previously would have skewed a starting pitcher’s ERA.
The advancements in technology have revealed the need for newer, more sophisticated metrics for ERA to remain relevant. This has led to the creation of advanced ERA estimators like FIP (Fielding Independent Pitching), xFIP (expected FIP), and SIERA (Skill-Interactive ERA), allowing for a deeper analysis by factoring in elements like ballpark effects and defense-independent outcomes. The use of such complex calculations—often embodied in predictive models—complements traditional ERA, offering a broader perspective on a pitcher’s true talent level. The 2019 MLB season saw the introduction of Automated Ball-Strike Systems (ABS), sometimes known as robot umps, in some minor league games, hinting at a future where technology might further suppress ERA fluctuations by standardizing strike zones. The evolution of ERA amidst this statistical boom is a testimony to baseball’s resilience and willingness to adapt, steering toward a future that honors the legacy of the game while embracing innovation.
The Antique ERA Calculation Methods in Baseball article takes us back to the origins, exploring the very first techniques used to quantify a pitcher’s performance. It offers a nostalgic look at how baseball’s statistical landscape has grown from these early calculations.
Sabermetrics Takeover: Advanced Metrics Revolution
The sabermetrics takeover in baseball is not merely an evolution; it’s a redefinition of how the game is played and understood. Data-driven insights have ushered in a metrics revolution, where advanced statistics have become as integral to baseball as bats and balls. In 2003, the publication of Michael Lewis’s “Moneyball”, which chronicled the Oakland Athletics’ analytical approach to team assembly, brought sabermetrics to the mainstream. The A’s success with a limited budget by focusing on on-base percentage (OBP) and slugging percentage (SLG) over traditional metrics like batting average, emphatically demonstrated the practical applications of advanced metrics. This approach, underpinning the philosophy of sabermetrics, has fundamentally altered talent evaluation and strategic decision-making in baseball.
The revolution extends beyond just recruitment and into the game’s strategy, with sabermetricians introducing a slew of novel metrics. WAR (Wins Above Replacement) effectively summarizes a player’s total contributions to their team, while wOBA (weighted On-Base Average) and wRC+ (weighted Runs Created Plus) provide nuanced views of a player’s offensive value. By quantifying and aggregating players’ offensive, defensive, and base-running skills, WAR offers a single number that expresses a player’s worth, greatly simplifying comparisons across positions and eras. Benchmarks like a WAR of 8.0, often indicating an MVP-caliber season, or a wRC+ of 100, signifying a league-average offensive player, are now commonly used in evaluating performance.
Simultaneously, the adoption of Statcast technology across MLB ballparks has given rise to entirely new data points. Metrics such as exit velocity, barrel rate, and launch angle are now analyzed to evaluate a batter’s potential beyond traditional hitting statistics. Pitchers are scrutinized through measures of spin rate and pitch movement, with higher spin rates often correlating with better pitch effectiveness. Visualizations and heat maps generated from Statcast data allow teams to optimize defensive alignments and pitching strategies in a way that was impossible before. As of the 2020 MLB season, Statcast tracked over 700,000 pitches, providing a wealth of data to dissect and analyze. The sabermetrics takeover embodies the spirit of innovation in baseball, continuously reshaping the game’s analytically minded future while enriching the narrative of America’s pastime.
The Dawn of Pitcher Context Era: Adjusted ERA+
As the language of pitching statistics matures, Adjusted ERA+ emerges as a pivotal metric offering a more comprehensive view of a pitcher’s performance. Unlike traditional ERA, which provides a raw score of a pitcher’s runs allowed on average per nine innings, Adjusted ERA+ accounts for the external factors that can skew those numbers, such as the quality of defense behind the pitcher and ballpark dimensions. This normalization allows us to compare players from different teams and eras more equitably. A figure of 100 represents a league-average ERA+, with numbers above 100 indicating better-than-average performance and Vice versa.
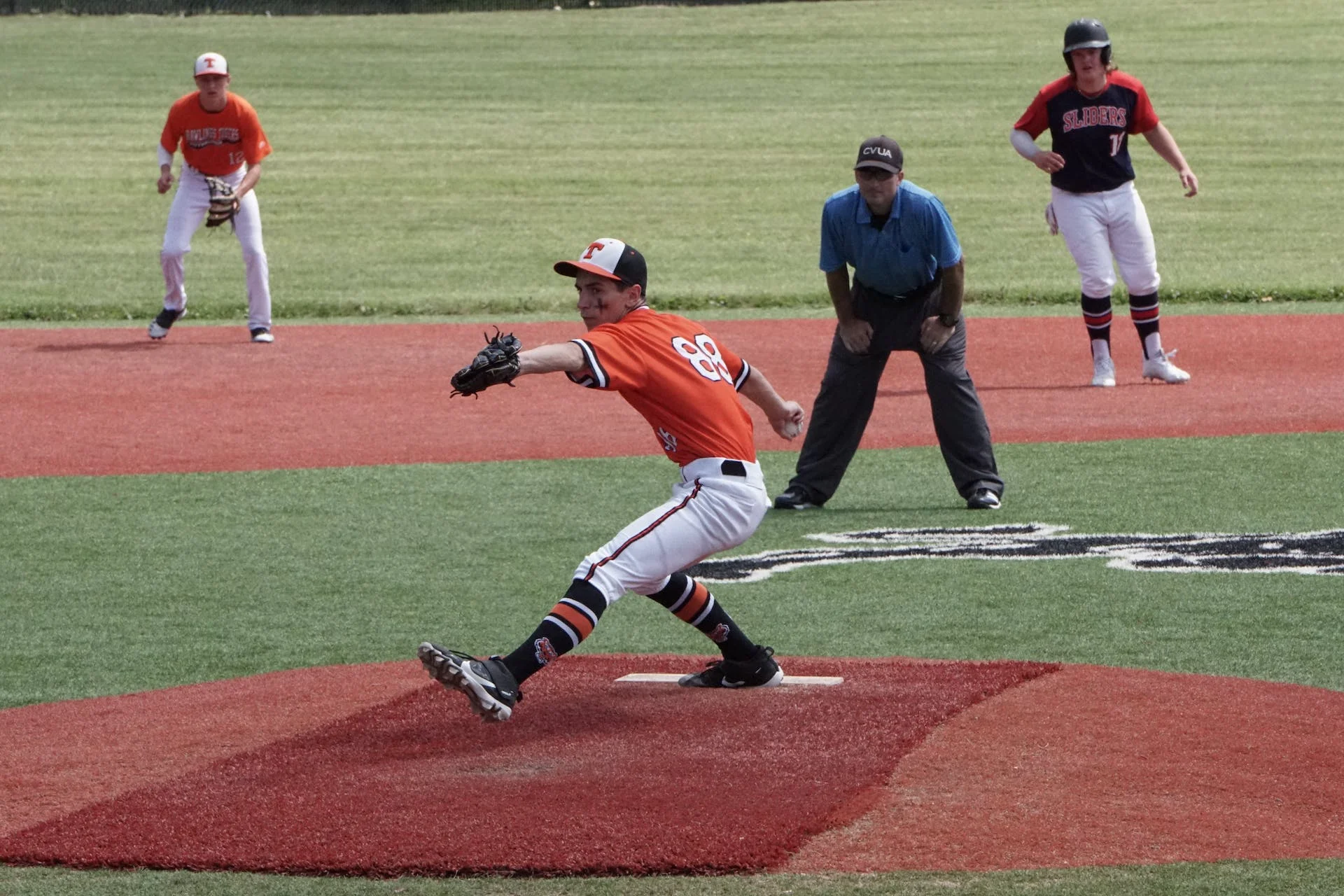
The inception of Adjusted ERA+ leverages the extensive data now at our disposal. It’s crafted to paint a more accurate picture by standardizing ERA across the league’s varying park factors. Notoriously hitter-friendly stadiums like Coors Field in Denver, with an altitude-aided atmosphere that can turn pop-ups into homers, skew pitchers’ ERAs higher. Meanwhile, expansive outfields like those at Oracle Park in San Francisco can pad a pitcher’s statistics unfavorably. Adjusted ERA+ compensates for these disparities, providing context by benchmarking a pitcher’s ERA against the league average—adjusted for park effects and the era in which they play. A score above 100 signifies that the pitcher is above league average after the adjustment.
Moreover, list-style evaluations have elevated Adjusted ERA+ as a quick-reference tool for assessing career-long performance. Rankings are compiled, spotlighting pitchers who consistently outperform the league average, a coveted trait among franchise players. For example, legends like Pedro Martínez, who boasts a career Adjusted ERA+ of 154, and Clayton Kershaw, hovering around 157, emphasize the metric’s utility in showcasing elite performance. Career benchmarks, such as Mariano Rivera‘s Adjusted ERA+ of 205, clearly demonstrate a pitcher’s dominance over contemporaries. This number indicates that Rivera’s adjusted ERA is more than twice as good as the league average during his tenure—a staggering feat. By considering Adjusted ERA+, franchises, analysts, and fans gain a refined lens through which to view and appreciate the artistry of pitching in Major League Baseball.
Contemporary Methods: FIP and xFIP Innovations
Following the trend of advanced baseball metrics, Fielding Independent Pitching (FIP) breaks new ground by focusing solely on a pitcher’s direct contributions: strikeouts, unintentional walks, hit-by-pitches, and home runs. This innovative stat strips away the variables he cannot control, such as the quality of the fielder’s play, to provide a more isolated assessment of his performance. By using a formula that weights these factors appropriately, FIP gives a more stabilized measure, a boon in predictive analytics. The reliability of FIP manifests in its capacity to foresee a pitcher’s future ERA trends more accurately than their current ERA. It’s predicated on the idea that whilst pitchers have limited control over batted balls in play, they have near-complete control over strikeouts, walks, and home runs.
Pushing the envelope further, xFIP (expected Fielding Independent Pitching) builds on FIP’s foundation but introduces a normalization of home run rates to the league average. The distinction lies in xFIP’s assumption that pitchers exhibit limited control over their home run rates in the long run, treating fly balls as a more consistent indicator of a pitcher’s skill set. Thus, xFIP adjusts a pitcher’s home run rate to what it ‘should’ be based on fly-ball rates, a revelation for those evaluating pitchers with aberrant home run-to-fly-ball ratios. This forward-thinking approach offers an even keener predictive edge than FIP, attempting to strip away the noise and nudge closer to the pitcher’s true talent level.
To delve into the numbers, pitchers with a lower xFIP are often underrated, while those with a higher xFIP may be overvalued. Take, for instance, a pitcher with an xFIP of 3.80 in the context of a league-wide FIP of 4.00 – this suggests expected performance better than the league norm, all else being equal. Rankings utilizing FIP and xFIP aid in uncovering hidden gems in the pitching market and potentially forecast breakout or decline phases for seasoned pitchers. It’s crystal clear that the sabermetrics era has evolved from raw data analysis into a nuanced dissection of a pitcher’s arsenal, opening a trove of meticulously detailed player value assessments.
ERA Precision: The Quest for the Perfect Pitching Stat
The relentless pursuit of baseball analytics ushers in the era geared toward perfecting pitcher evaluation metrics. Sabermetricians tirelessly mine decades of gameplay statistics, blending traditional data with cutting-edge technology to craft the ultimate pitching stat — one that encapsulates a pitcher’s prowess with unmatched accuracy. At the epicenter of this quest is the hunt for a statistic or set of statistics that converge on the distillate of pitching greatness. This number must be resilient to the variances of defense, ballparks, and weather —unbiased and refined to the essence of individual performance.
The ideal pitching stat would be a composite of several metrics, synthesizing the insights gleaned from Adjusted ERA+, FIP, and xFIP. For instance, WHIP (Walks and Hits per Inning Pitched) offers a glance into a pitcher’s ability to keep baserunners at bay, while Strikeout-to-Walk Ratio (K/BB) underscores a pitcher’s control and power. Combining such metrics could yield a multi-faceted evaluation tool; items in this statistical buffet might also include:
- Quality Start Percentage (QS%): The frequency with which a pitcher executes starts of six or more innings while allowing three earned runs or fewer.
- Win Probability Added (WPA): A reflection of a pitcher’s influence on the game’s outcome, measuring the swing in win probability with each pitch.
- Run Expectancy of the 24 base/out states: A nuanced measure of a pitcher’s effectiveness in various game situations.
The breakthrough stat would also incorporate contemporary tech marvels like Statcast, which provides granular data on pitch velocity, spin rate, and launch angles. These numbers offer a glimpse into a pitcher’s ability to induce weak contact or overpower hitters with sheer velocity. Such metrics could lead to an era where scouting reports become as sophisticated as the arsenal of pitches in a Cy Young contender’s repertoire. The coveted pitching stat may even leverage machine learning to predict how a pitcher’s performance would transfer across different team lineups and stadium environments, providing a truly comprehensive assessment. This evolution of analytics will not just reflect past performance but may also predict future success with a degree of precision that was once the stuff of fantasy. As this search continues, sabermetrics reaffirms its commitment to quantifying the mastery of the mound, moving closer to answering what truly makes a pitcher great.
Conclusion
In baseball’s analytical journey, seven historical ERA calculation methods have been prominent. From rudimentary formulas to savvy tech integration, each evolved from its predecessors, illuminating a pitcher’s impact with a clearer perspective. This voyage through numbers has enriched our understanding of the mound’s masters, merging tradition with innovation. Sabermetrics hasn’t just tabulated; it has narrated the game’s rich legacy, recasting pitcher prowess into legible lore for fans and analysts alike. The quest for perfecting pitcher evaluation is a testament to baseball’s enduring love affair with statistics and the timeless quest to quantify greatness.
Reflecting on the legacy of ERA in baseball, ERA Computation in Baseball: Past Methods delves into the historical computation techniques. This narrative highlights how past methods laid the groundwork for the sophisticated analytics that define today’s game, showcasing the evolution from simplicity to complexity.
FAQ’s
What were early methods for calculating ERA?
Early ERA calculation methods in baseball involved basic formulas dividing earned runs by innings pitched, lacking sophistication compared to modern metrics. These methods provided a foundational understanding of pitcher effectiveness but were limited in accounting for nuances in game situations and defensive support.
How did baseball historically calculate ERA?
Historical approaches to calculating baseball ERA relied on simple formulas dividing earned runs by innings pitched, with variations in interpretation and recording across leagues. These methods served as early benchmarks for pitcher performance evaluation, laying the groundwork for more refined ERA calculation techniques in modern baseball analytics.
Are there notable changes in ERA calculation?
Notable changes in historical ERA calculation include adjustments in defining earned runs, varying interpretations of game situations, and evolving recording standards across baseball leagues. These changes reflect the ongoing refinement and adaptation of ERA calculation methods to better capture pitcher effectiveness and game dynamics over time.
Who pioneered early ERA calculation techniques?
Pioneers of early ERA calculation techniques include baseball statisticians and analysts who sought to quantify pitcher performance using rudimentary metrics. Figures like Allan Roth and Earnshaw Cook contributed to the development of ERA calculation methods, paving the way for advancements in baseball analytics and statistical evaluation of player performance.
What historical factors influenced ERA calculations?
Factors influencing historical ERA calculations include changes in scoring rules, defensive strategies, and recording standards across baseball leagues. Additionally, advancements in statistical analysis and data collection techniques have shaped the evolution of ERA calculation methods, enhancing their accuracy and relevance in evaluating pitcher effectiveness over time.
How has ERA calculation evolved?
The evolution of ERA calculation over time reflects advancements in statistical analysis, data collection methods, and understanding of game dynamics. From early simplistic formulas to modern sophisticated metrics, ERA calculation has undergone continuous refinement to provide more accurate and comprehensive insights into pitcher performance in baseball analytics.